1. Science fiction merges into reality
When Isaac Asimov introduced the world to his famous Three Laws of Robotics in the short story ‘Runaround,’ he might not have envisioned just how closely the world, eighty years later, would resemble his sci-fi dreams. Today, we inhabit a world permeated with Artificial Intelligence (AI). AI systems have surpassed Asimov’s imagination in many ways. For example, at home, personal AI assistants can not only schedule your day but also recommend entertainment based on your mood. In the workplace, various AI analytics tools enhance industry efficiency and deepen scientific understanding. Even in the realm of art, AI is assisting countless artists in creating innovative works, challenging our traditional notions of creativity.
But this is just the tip of the iceberg.
AI weather models1 now predict the weather for us. They outperform traditional numerical forecasting methods, which represent millennia of human experience, in both accuracy and speed—by a factor of ten thousand. AI teaching platforms are providing personalized guidance based on students’ learning habits and progress2, making education more effective and inclusive. AI medical models are also aiding doctors in diagnosing rare diseases3, cancer4, and neurodegenerative disorders5 more accurately and quickly. In some cases, they even outperform human experts.
However, these advancements raise a question:
Do these advanced AI systems represent humanity’s ultimate vision of Artificial General Intelligence (AGI), or ‘super-human level intelligence’?
Ever since ‘Artificial Intelligence’ was first proposed at the Dartmouth Conference in 1956, achieving human-level intelligence has been a paramount goal in the AI field. In the first half of this year, mainstream researchers6 posited that AI models, particularly large language models, have demonstrated ‘sparks of AGI’. This suggests that AGI has transitioned from being a philosophical speculation to becoming a future possibility. However, opinions on AGI vary widely, and the occasional erratic behavior of large language models raises questions about the feasibility of true AGI. Given this context, several key questions arise: What is our exact goal for AGI? How close are we to achieving true AGI? And how can we forecast AGI’s social impact and evaluate its potential risks?
To answer these questions, we must first define AGI accurately and operationally, beyond the imaginative scenarios presented in science fiction works.
2. Attempting to Define AGI
The attempt to define the concept of Artificial General Intelligence (AGI) can be traced back to the famous ‘Turing Test’7 of the 1950s. In this test, a human communicates with an unknown entity using text and must determine whether the entity is a machine or a human. Turing’s insight was that a machine’s ability to ‘think’ does not depend on its thought process but rather on its demonstrated capability. However, this test is often criticized for not adequately reflecting true intelligence levels, as it relies on the human evaluator’s subjective judgment, which can be easily deceived.
Subsequently, John Searle, in his famous ‘Chinese Room’8 thought experiment, viewed AGI as a strong AI system with ‘consciousness.’ While the idea of linking AI with ‘consciousness’ is intriguing, it remains more a philosophical topic than a scientifically verifiable concept, given the challenges in defining ‘consciousness’ itself.
When Mark Gubrud first proposed the concept of AGI in 1997, he likened it to the human brain—a system capable of surpassing the brain in complexity and speed, acquiring and internalizing knowledge, and being used for purposes requiring human intelligence. However, modern AI systems, such as those based on the Transformer model, have a limited connection to human brain structure and learning patterns.
More recently, in the early 21st century, when DeepMind co-founder Shane Legg popularized the concept of AGI among computer scientists9, he defined AGI as a machine intelligence capable of human-like performance in cognitive tasks. Additionally, this definition does not specify the types of tasks or the standard for ‘human-like’ performance.
Additionally, recent attempts have been made to define AGI in terms of learning tasks or metacognitive abilities (deemed insufficient), economic value (considered unnecessary and insufficient), and flexibility (deemed insufficient), among other aspects. However, each of these attempts has its own limitations. This year, some influential scientists10 proposed that current state-of-the-art (SOTA) large language models are already AGI, as they perform sufficiently well in a variety of tasks and are versatile enough; however, beyond versatility, true AGI must also possess sufficiently reliable performance.
In essence, these attempts seek to define a ‘critical’ or ‘ultimate’ state of AI development. However, the journey to the pinnacle of AGI is likely not a single peak, but rather a complex plateau with layered landscapes and intricate terrain.
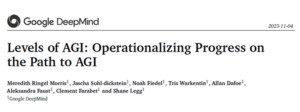
Fig. 1. Original Research Paper, from Reference 12.
Recently, Shane Legg and the DeepMind team summarized historical definitions and proposed their framework for defining AGI12.
3. From Theory to Practice: The Six Principles of Defining AGI
From these past attempts to define AGI, researchers have identified some common features and extracted six essential principles that any definition of AGI must meet:
1.Focus on Capabilities, not Processes. The definition of AGI should focus on the tasks it can accomplish, rather than the specific ways these tasks are completed. This means that achieving AGI does not imply that the system must think or understand in a human-like manner.
2.Focus on Generality and Performance. AGI should be capable of performing a wide range of tasks (breadth, generality) and achieve a certain level of performance in these tasks (depth, performance).
3.Focus on Cognitive and Metacognitive Tasks. AGI must be capable of handling cognitive tasks (such as problem-solving, creative thinking) while also possessing metacognitive abilities (like learning new skills, or knowing when to ask for human help).
4.Focus on Potential, not Deployment. When assessing AGI, the focus should be on its potential capabilities, rather than its current practical applications (which depend on real-world deployment).
5.Focus on Ecological Validity. AGI should be able to solve real-world problems, with its value lying in addressing the complex and diverse challenges of everyday life.
6.Focus on the Path to AGI, not a Single Endpoint. Just as establishing a set of standards for autonomous driving is beneficial for policy-making and progress in autonomous vehicles, defining AGI’s standards at different ‘levels’ is useful. The development of AGI is akin to a long journey, where the ultimate goal may not be a fixed point, but an evolving process.
4. Understanding AGI: Defining Levels
From this perspective, researchers have identified ‘Performance’ and ‘Generality’ as the two core dimensions for assessing the degree of AGI in AI systems. ‘Performance’ measures the ‘depth’ of an AI system’s capabilities, namely, how the system’s performance compares to human performance in given tasks. ‘Generality’ measures the ‘breadth’ of an AI system’s capabilities, specifically, the range of tasks the AI system can solve. Considering non-AI computing systems, such as the most basic calculators, as the starting point of AI development. In contrast, an AI system that surpasses all human performance, such as AlphaZero in chess11, represents the ultimate form of AI. Based on this, AI’s performance development can be divided into six stages: No AI, Emerging, Competent, Expert, Virtuoso, and Superhuman. Simultaneously, researchers classify AI systems into ‘Specialist’ systems focused on specific tasks and ‘Generalist’ systems capable of handling various tasks. This classification allows for the following categorization of existing AI systems:
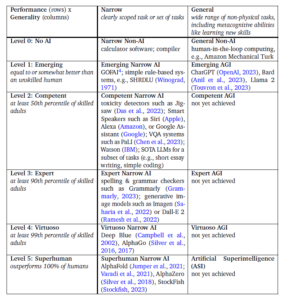
Fig. 2. A leveled, matrixed approach toward classifying systems on the path to AGI based on depth (performance) and breadth (generality) of capabilities. Example systems in each cell are approximations based on current descriptions in the literature or experiences interacting with deployed systems. Figure source: Reference 12.
5. Testing AGI Using This Framework
AGI testing is a crucial step in evaluating the comprehensive capabilities of AI. The six principles mentioned above provide a comprehensive framework for understanding and measuring AGI. Among these principles, ‘universality’ and ‘performance’ guide researchers in constructing a layered ontological framework, allowing a detailed exploration into the breadth and depth of AI’s capabilities.
In AGI testing, the performance dimension is relatively straightforward: we can evaluate it by comparing the AI system’s performance with that of humans in the same tasks. This is similar to evaluating a Go player not just by the number of games won, but also by the skill levels of the opponents defeated.
The dimension of generality is more challenging. It requires answering a critical question: What range of tasks must an AI system master to be considered sufficiently broad? Consider an AI capable of mathematical reasoning, understanding natural language, and composing music. While this demonstrates a broad range of abilities, the question remains: is this sufficiently general? It’s akin to identifying the most representative stars in the vast universe of skills – a task that is both broad and significant. Researchers believe that more study is needed in this area.
It’s noteworthy that when assessing AGI’s performance, the tests used should be as close to real-world applications as possible. For instance, comparing an autonomous vehicle with a human driver who does not use any modern AI tools is not very convincing. A more meaningful comparison would be with human drivers who already utilize some driving assistance technologies.
Furthermore, AGI benchmark tests may include open-ended and interactive tasks requiring qualitative assessment (in other words, human subjective evaluation). This is like judging an art competition where the judges consider not only the technical level of the works but also their creativity and expressiveness. For example, we might ask an AI system to interpret a literary work or compose a poem, tasks that test not just the AI’s technical ability but also its innovativeness and understanding of human emotions.
Finally, building a benchmark test for AGI is an iterative and improving process. The benchmark itself must also be constantly updated to accommodate the various tasks AGI might complete, as we can never exhaustively list all the tasks AGI should perform. This is not just for measuring AGI’s current capabilities but also to inspire its evolution toward higher intelligence. This process presents a technical challenge and reflects our evolving understanding of intelligence. Importantly, it will also guide the future direction of technological development.
6. Other Research Directions
In addition to constructing benchmark tests for AGI, several other research directions are crucial for advancing towards AGI.
Alignment
The goal of alignment is to ensure that the behavior of AI systems aligns with human interests and values. This is crucial for preventing unpredictable consequences and potential harms posed by AI systems. At this year’s NeurIPS and the 2024 ICLR, there was an abundance of papers and research submissions focusing on this area, underscoring its importance. These studies concentrate not only on theoretical and technical progress but also on corresponding governance measures to ensure AI adheres to human ethics and values in complex and dynamic environments. As AI systems increasingly integrate with society, the value of alignment research becomes ever more apparent.
Mechanistic Interpretability
Research related to mechanistic interpretability aims to reveal how AI systems make decisions and the mechanisms behind these decisions. Enhancing our understanding of the inner workings of AI systems allows us to more effectively monitor and guide their behavior, ensuring alignment with human expectations and standards.
Interdisciplinary Collaboration
Interdisciplinary collaboration also plays a vital role in AGI research. It necessitates collaboration among experts from diverse fields, including computer science, philosophy, psychology, neuroscience, law, sociology, and political science. This collaboration is instrumental in comprehensively understanding the potential impacts of AGI and in offering innovative solutions to its associated challenges.
7. The Multifaceted Nature of Risks: From Existential to Extreme Risks
When discussing AGI, various concepts of risk are often mentioned, from ‘x-risk’ (existential risk) to various types of extreme risks. Existential risks refer to those that threaten human survival, while extreme risks cover a range of dangers that could severely impact society. The multifaceted nature of these risks arouses our curiosity about the future and simultaneously reminds us to proceed with caution, as new challenges and opportunities may lurk around every corner.
AGI Levels and Risk Assessment
By defining a framework of AGI levels, we can more clearly see the risks associated with different levels of AGI, rather than assessing it as a single event. Under this framework, policymakers can identify and prioritize the risks brought by the current development stage. For example, expert-level AGI might lead to job displacement due to machine intelligence, causing economic turbulence, but it might also reduce some issues seen at lower AGI levels, such as risks of improper task execution. This process is akin to balancing and adjusting while climbing a technological peak, ensuring that humanity does not compromise safety in the pursuit of advancements.
However, it must be acknowledged that the pace of technological iteration, especially in the AGI field, may outstrip existing regulations and diplomatic policies. It’s as if we’re driving a high-speed train with road rules still stuck in the era of horse-drawn carriages. This presents more complex impacts. For instance, the first country to achieve ASI (Artificial Superintelligence) might gain significant geopolitical and military advantages, potentially leading to a series of risks and challenges.
Therefore, researchers believe that AGI benchmark testing should not only measure AI capabilities but also assess potential risks. This includes how to handle dangerous capabilities that AGI might possess, such as deception and suggestive persuasion. When nurturing an all-capable entity, we must teach it not only knowledge and skills but also how to use these abilities responsibly and ethically. For instance, Anthropic’s responsible expansion policy12 illustrates a method of balancing innovation and safety, by setting different risk levels and preventive measures for AI systems at various stages of development.
Autonomy and Risk: The Art of Design
In considering AGI applications, we also need to think about how they interact with humans. Returning to the example of autonomous vehicles, fully autonomous vehicles (Level 5) require no human intervention, while for lower autonomy levels (such as Level 2), human drivers still play an important monitoring and control role. In the table below, researchers describe the paradigm of human-machine interaction through six levels of autonomy. In this paradigm, the level of an AI system’s autonomy is directly linked to its composite capabilities. This means that as AI systems become more advanced and autonomous, they can offer more diverse interaction modes, also potentially introducing different types of risks.
Different levels of autonomy represent different ways of collaboration between humans and AI. Sometimes, even if higher levels of autonomy are technically feasible, choosing lower levels may still be wiser for educational, entertainment, or safety reasons. For example, in the field of autonomous driving, even if full automation is possible, we might prefer to involve human drivers to ensure safety and adaptability. Considering AGI systems in real-world scenarios helps us better understand the relationship between model progress and the evolution of AI interaction paradigms. This consideration helps predict the direction of AI system development and guides us in designing these systems to ensure that advancements serve human needs without sacrificing safety.
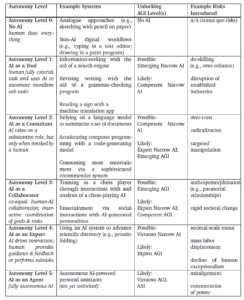
Fig. 3. Levels of AGI Achievement Across Different Autonomy Levels and Their Associated Potential Risks. Figure source: Reference 12.
8. OpenAI’s Perspective: AI Development and Its Impact on Society
Earlier this year, OpenAI’s CEO, Sam Altman, discussed the potential impacts of AGI on society, technology, and ethics in his blog post titled ‘Planning for AGI and Beyond’12. He emphasized the necessity of careful planning and responsible development.
The article suggests that AGI has the potential to greatly enhance the quality of human life by increasing resource richness, driving global economic growth, and aiding in the discovery of new scientific knowledge. This signifies not just a tremendous boost in intelligence but also a notable expansion of human creativity and imagination. However, the emergence of AGI could also bring serious risks of misuse, the possibility of unexpected extreme events, and drastic upheaval in social structures. Therefore, OpenAI advocates for AGI developers and society as a whole to find appropriate ways to develop and utilize this technology, ensuring alignment with core human values and equitable sharing of its benefits.
Furthermore, OpenAI believes that the advent of AGI is merely a milestone in the development of intelligence, and that the advancement of AI may continue for a considerable time in the future. OpenAI envisions a safe development of AGI, advocating for a gradual transition over a shorter period to allow society adequate time to adapt and adjust.
Despite the unpredictability of the future, OpenAI articulated several key principles that they care most about:
OpenAI aspires for AGI to maximize the flourishing of humanity in the universe.They wish for the benefits, access, and governance of AGI to be shared broadly and equitably, successfully addressing significant risks.
To achieve this, OpenAI supports a gradual transition in the short term, focusing on creating increasingly aligned models, and advocates for engaging in global conversations in the long term. These discussions should cover how to govern these systems, how to equitably distribute the benefits they generate, and how to fairly share access. This transition to a world with superintelligence may represent the most important, hopeful, yet fearful era in human history, with no guaranteed outcomes. However, given the extremely high risks and rewards, humanity needs to unite and ensure that AGI unfolds in the future world in a way that is most beneficial to humankind.
9. Conclusion: The Necessity of Steady Progress
Overall, the advancement of AGI represents not only technological innovation but also a reimagining of future human-machine interactions. As we delve into the uncharted territories of AGI, robust and orderly development becomes crucial. This is essential to ensure that humanity does not overlook potential risks while pursuing technological advancements. Understanding the different development levels of AGI systems and their corresponding autonomy levels allows us to continually assess and adjust our pace. This approach better prepares us for future challenges and ensures that the development of these technologies benefits humanity safely and responsibly.