In the field of neuroscience, brain-computer interfaces (BCIs) are recognized as a critical frontier of innovation. This February, “Nature Electronics” named them the Technology of the Year for 2023, emphasizing this moment as pivotal for reflecting on the direction of BCI advancements. In July, the United Nations Educational, Scientific and Cultural Organization (UNESCO) published a report focusing on neurotechnology and its implications, highlighting BCIs [1].
BCI technology continues to advance rapidly, breaking new ground. A “Nature Neuroscience” study from October introduced a concept called “precognition without motion.” This technique involves using functional ultrasound (fUS) neuroimaging to interpret the movement intentions of monkeys without requiring any physical movement or implanting electrodes [2]. The research, led by Professor Richard Andersen of the California Institute of Technology and director of Tianqiao & Chrissy Chen Institute, represents a significant stride in the field.
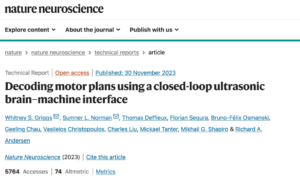
Figure Source: Paper Cover, Reference 2.
In this research, the researchers worked with two rhesus monkeys trained to use a brain-machine interface (BMI). These monkeys could control up to eight motion directions in real-time using their thoughts, observed through fUS data collected from their posterior parietal cortex during eye and hand movement tasks. The researchers developed a method allowing the BMI to be pre-trained with data from previous sessions, which enabled immediate control in future sessions and reduced the need for recalibration. This innovation opens new possibilities in brain-machine technology, offering insights into “ultrasound telepathy.”
To explore the practical application of fUS in BMI, the researchers first decoded two motion directions in a real-time, closed-loop setting as monkeys performed eye movement tasks from memory, either left or right. After 100 successful trials, the study progressed to a closed-loop decoding phase. Here, the monkeys controlled the task direction solely through their intent, receiving feedback after each attempt to indicate if the BMI successfully predicted the direction. Following 55 training trials, the decoder’s accuracy improved significantly, achieving 82% by the 114th trial.
Addressing the challenge of recalibration over multiple sessions, the team achieved stable decoding performance with the fUS-BMI by employing a pre-training strategy. They compared performance using data from only the current session to that pre-trained with data from previous sessions, demonstrating significant enhancements in performance and reductions in training time.
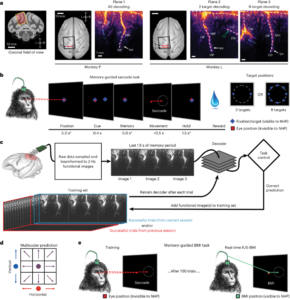
Figure Source: Pre-training Process for Cross-Session Decoding, Reference 2.
After addressing recalibration across time zones and ensuring the performance of online closed-loop decoding, researchers are now seeking to further enhance fUS-BMI by enabling real-time decoding of movement in eight directions. Initially, they independently predicted the vertical (up, middle, down) and horizontal (left, middle, right) components of movement intention, later combining these into a final forecast (e.g., upward and to the right). This approach aligns with the PPC neurons’ response characteristics, exhibiting similar responses for adjacent motion directions and distinct ones for larger angular separations. Therefore, this multi-encoder strategy considers the neural representation similarities between adjacent directions instead of treating the eight directions as entirely distinct categories. The decoder reached a notable accuracy (significantly above the 12.5% random level) after 86 training trials and stabilized at an accuracy of 34-37%. Further retesting revealed that pre-training significantly reduced the number of trials required for effective decoding.
Thanks to the fUS technology, researchers were able to record activities in multiple functional brain regions, such as those encoding hand and eye movements. To validate this, they replicated the aforementioned training and decoding process in a manual task and also achieved favorable results.
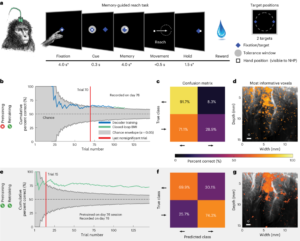
Figure Source: Oculomotor and Manual Task Experiment Flow, Reference 2.
1. The Principles of Ultrasound Mind Reading
The most direct aim of brain research is to comprehend, interpret, and ultimately achieve autonomous control of the brain. Brain-computer interfaces (BCIs) facilitate this by providing a direct communication link between the brain and external devices, enabling the recording, decoding, and stimulation of neural activity. Commonly, neural signals propagate as electrical impulses along neurons, with the most direct measurement being through electrodes that record local field potentials (like ECoG) or signals that pass through the scalp (like EEG). Beyond these direct methods, there are numerous indirect ways to measure neural activity, such as functional Magnetic Resonance Imaging (fMRI) and calcium ion (Ca2+) imaging. The ultrasonic signals employed in this study represent such an indirect method, having been used in brain imaging for just over a decade.
Functionally, fUS mirrors fMRI in its reliance on the neurovascular coupling effect. It uses ultrasonic waves to image the hemodynamics parameters (like blood volume) in the brain’s small arteries and capillaries, indirectly inferring neural activity at approximately 1 Hz. The locational information is based on the pulse wave Doppler effect, interpreting the motion information of reflected targets from the stationary emission source and moving reflection target by analyzing the reflected sound from the ultrasound waves. To achieve deeper imaging depths, fUS must use extremely short ultrasound pulses, making direct frequency variation measurement challenging. However, by sending multiple pulses and integrating the reflected signals, it’s possible to indirectly calculate short-term delays in frequency—this is the pulse wave Doppler effect.
After collecting imaging data, the neuronal information must undergo a critical step: filtering. Blood cells are surrounded by moving brain tissue, which also generates Doppler signals. The process of separating these blood and tissue signals is crucial. Because brain tissue movement from cardiac, respiratory, and behavioral activities is typically slower, it generates Doppler signals with lower absolute frequencies than blood. High-pass filters can eliminate these tissue signals, retaining only the blood signals. More advanced filtering techniques can further optimize this separation, making efficient filtering particularly crucial for recordings in awake states [3].
2. The annals of “Ultrasound Mind Reading”
The bulk of fUS research to date has occurred within rodent models [4]. Initially, researchers were limited to experiments on anesthetized rats with fixed heads. However, advances in clinical techniques now allow for the collection of fUS signals in awake, head-fixed mice through well-tolerated chronic cranial windows [5]. In rodents, fUS signals were primarily employed to assess the feasibility of the technology. Only recently have studies on awake, head-fixed mice begun to emphasize the relationship between whole-brain imaging and behaviors or neural circuit manipulations [6]. Alongside the development of fUS fixed imaging, there has been an expansion of its application across different species, demonstrating its broad applicability to multiple species by merely adjusting frequencies to accommodate various brain volumes.
One of the distinct advantages of fUS is its ability to track whole-brain activity in freely moving rodents. This technique requires a cranial window and bracket implanted on the animal’s head. During experiments, researchers insert an ultrasound probe, connected via cable to the scanner, as the animal runs or performs tasks. The development of free-moving imaging technology has benefited from the fabrication of miniature linear ultrasound probes. These micro probes have a rigid cable and can image one plane at a time, offering a pathway to observe deeper brain activity during more natural tasks than those performed while head-fixed. After demonstrating the technology’s feasibility [6], free-moving fUS in rats has primarily been used for systemic-level behavioral state studies, such as movement or sleep, which occur more naturally without constraints [7]. Furthermore, combining fUS imaging with concurrent electrophysiological recordings can correlate large-scale hemodynamic patterns with specific regional neuronal activities.
Beyond rodent models, fUS is also appealing for non-human primate research. While technically similar, it’s adapted for the unique brain structures of primates, such as monkeys, by using lower frequencies for deeper imaging. For instance, a recent study utilized fUS for detailed investigations of the deep visual cortex in awake non-human primates [9]. Additionally, fUS has been used in macaques for imaging memory-guided motor tasks, considered the first work decoding primate brain motor plans with fUS. Despite the lower temporal resolution due to vascular signal interference, this lays an important foundation for using deep brain signals to control external mechanical devices. Since the publication of this work, scientists have continuously broken new ground in clinical applications and data analysis.
This year, a significant breakthrough in signal processing and data analysis for this technology came from Professor Richard Andersen’s team [2]. Around the same time, the authors published the first instance of using fUS to monitor brain activity in a fully intact adult human skull [10]. This breakthrough provides researchers a new avenue to acquire high-sensitivity, large-scale, high-resolution neural imaging without invasive entry into the human body. Using this technique, researchers achieved the first completely non-invasive mapping and decoding of cortical responses to finger movements, generating high-resolution (200 micrometers) and large-scale (50mm x 38mm) brain imaging. This research will deepen our understanding of brain activity, propel the development of neuroscience and brain-computer interface technologies, and offer innovative approaches to treating neurological diseases and BCI applications.
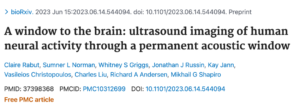
Figure Source: The first research to use fUS for monitoring brain activity in an adult human skull, Reference 10.
3. Comparison of “Ultrasound Mind Reading” and other signals
We can now better appreciate the distinctive features of functional Ultrasound (fUS) imaging. The differences mainly manifest in performance, invasiveness, coverage, spatiotemporal resolution, portability, and consistency in decoding over time.
Currently, the most advanced brain-machine interfaces utilizing subdural ECoG or invasive electrodes can decode over 15-60 words per minute, 29-90 characters per minute, and even individual finger movements with high accuracy.
Conversely, non-invasive EEG, another common technology for controlling BMIs, achieves 70-90% accuracy in binary classification of movement intentions but exhibits significant individual variability.
Turning our attention to fUS, it offers broad coverage and high spatial resolution (100 micrometers). With a 15.6MHz ultrasonic probe, it provides a large and deep field of view of 12.8 mm × 20 mm, reliably recording motion signals from multiple cortical areas and broadly distributed brain regions. Many brain-machine interface technologies are limited to recording from shallow cortical layers, a few millimeters deep (like ECoG, Utah arrays, and calcium imaging). However, in this study, researchers captured signals from the middle to deep layers of the LIP brain area, about 5-9 millimeters below the cortical surface. These are most informative for decoding eye movement, a feat other technologies cannot achieve.
While fUS performance is currently similar to EEG, it holds more potential for development compared to EEG, fNIRS, and fMRI. Previous research has found that the accuracy of decoding using fUS signals from the PPC area deteriorates rapidly as spatial resolution decreases, suggesting that other brain-machine interfaces with macro-scale spatial resolution will struggle to decode nuanced information within micro and meso-scale neural populations effectively.
fUS also benefits from easy repositioning and the ability to penetrate soft tissue. Invasive electrode arrays are typically implanted once and often placed suboptimally to avoid major blood vessels or due to poor positioning. Requiring additional surgery, repositioning these arrays is difficult. Tissue reactions can decrease the performance of subdural and intracranial chronic electrodes. With fUS, however, the ultrasound probe can be positioned, tested, and repositioned multiple times before locking in place, making it easier to locate and record from specific areas of interest. Theoretically, fUS can operate indefinitely through the dura mater, allowing chronic imaging over extended periods without signal degradation.
Despite being an emerging technology, fUS has significant potential. However, its limitations and constraints must also be acknowledged. It is subject to meso-scale neurovascular coupling (on the order of seconds), where the neurovascular response acts as a low-pass filter for each voxel signal, leading to a lower temporal resolution. Real-time delays of about 800 milliseconds occur with 2Hz fUS, hindering real-time imaging. Currently, technology permits only retrospective correlation analysis with previously acquired offline fUS data to approximate results with 100Hz fUS imaging.
Yet, when considering spatiotemporal resolution comprehensively, fUS retains unique advantages. It can detect neural hemodynamic signals within the brain, such as monitoring biomarkers for neuropsychiatric diseases. In some brain-machine interface applications, even with slower meso-scale neurovascular responses, it’s possible to extract signals related to the trajectory of intended movement.
4. The Future Direction of Ultrasound Mind Reading
So, what is the significance of this research? Simply put, researchers have demonstrated and validated the efficacy of an online, closed-loop functional Ultrasound Brain-Machine Interface (fUS-BMI), representing the cutting-edge of current advancements. They pinpoint two significant breakthroughs.
- Decoding More Movement Directions:
Building upon previous results decoding two directions of eye or hand movement, they have now successfully achieved real-time decoding of eight movement directions.
- Achieving Cross-Session Decoding Stability:
Intracranial electrode BMIs, like the Utah array, excel at sensing fast-changing (millisecond-level) neural activity from spatially localized regions (less than 1 cm), such as the motor M1 area and the visual V1 area, during behavior or stimulation. However, these intracranial electrodes struggle to track individual neurons over longer periods (e.g., between subsequent recording sessions), typically necessitating daily retraining of decoders. Similar challenges of neural group identification, including field-of-view shifts between experimental sessions, exist for ultrasound devices. The current study addresses these challenges by using an alignment method that stabilized image-based BMIs over a month or more. Even if retraining is necessary, it’s minimized from the same neurovascular groups.
This development is crucial, allowing researchers to easily align the previous day’s model with new data and begin decoding with minimal or no new training data. Compared to traditional manifold-based methods, the authors’ decoder alignment algorithm leverages fUS neuroimaging’s inherent spatial resolution and field-of-view to ensure decoder stability in an intuitive, replicable, and high-performance manner. They generated an image of the current session’s anatomy using a single fUS frame (~500 milliseconds) and aligned the previous session’s field of view to this single image. Notably, this requires no additional behavioral data for alignment.
As for how future improvements might be made in ultrasound imaging for brain-machine interfaces, the authors suggest several approaches. Regarding sampling areas, they recommend changing the placement of the head post and probe to allow imaging of a larger range of the LIP brain region. Additionally, in terms of imaging technology, while past focus has been on obtaining single-layer brain slice images, 3D volumetric imaging is a potential solution for more comprehensively capturing brain activity. However, due to bandwidth, memory, and computational limitations, high-quality, low-latency real-time 3D fUS imaging is not yet feasible. Yet, continual advancements in hardware and algorithms may soon realize 3D fUS-BMI.
Lastly, another path to enhanced performance might lie in using more advanced decoder models to replace the current linear decoders. Convolutional Neural Networks, designed to recognize image features, are robust to spatial perturbations common in fUS images, such as those related to breathing or heart rate. Moreover, Recurrent Neural Networks and Transformers models, using a process of “memory,” might be particularly adept at characterizing the temporal structure of fUS time-series data. However, these Artificial Neural Networks (ANNs) require more training data. Thus, the authors introduced a cross-session image alignment method, allowing previously recorded data to be compiled and organized into a large data corpus, potentially sufficient to train many ANNs. Beyond the data volume needed to train ANNs, recent studies also emphasize the additional challenges faced when training deep learning models for closed-loop motor BMI control, particularly avoiding overfitting to temporal structures in previously recorded data. While ANNs have not yet been used in current BMI experimental research, they hold great potential for future studies of more complex fUS-BMIs.
5. In Conclusion
As we ponder the interaction between the brain and machine, one inevitably wonders how far humanity can truly go. Reflecting on the step-by-step development of functional Ultrasound (fUS) enhances our understanding. Its recent thrilling research breakthroughs have shed light on the potential of this nascent technology.
Perhaps in the near future, we will witness it being more widely applied in humans to decode increasingly complex “thoughts.” At that point, it won’t be seen as a mysterious artifact from beyond but rather as a crystallization of human wisdom spanning medicine, engineering, mathematics, and various other fields.